Containing AI
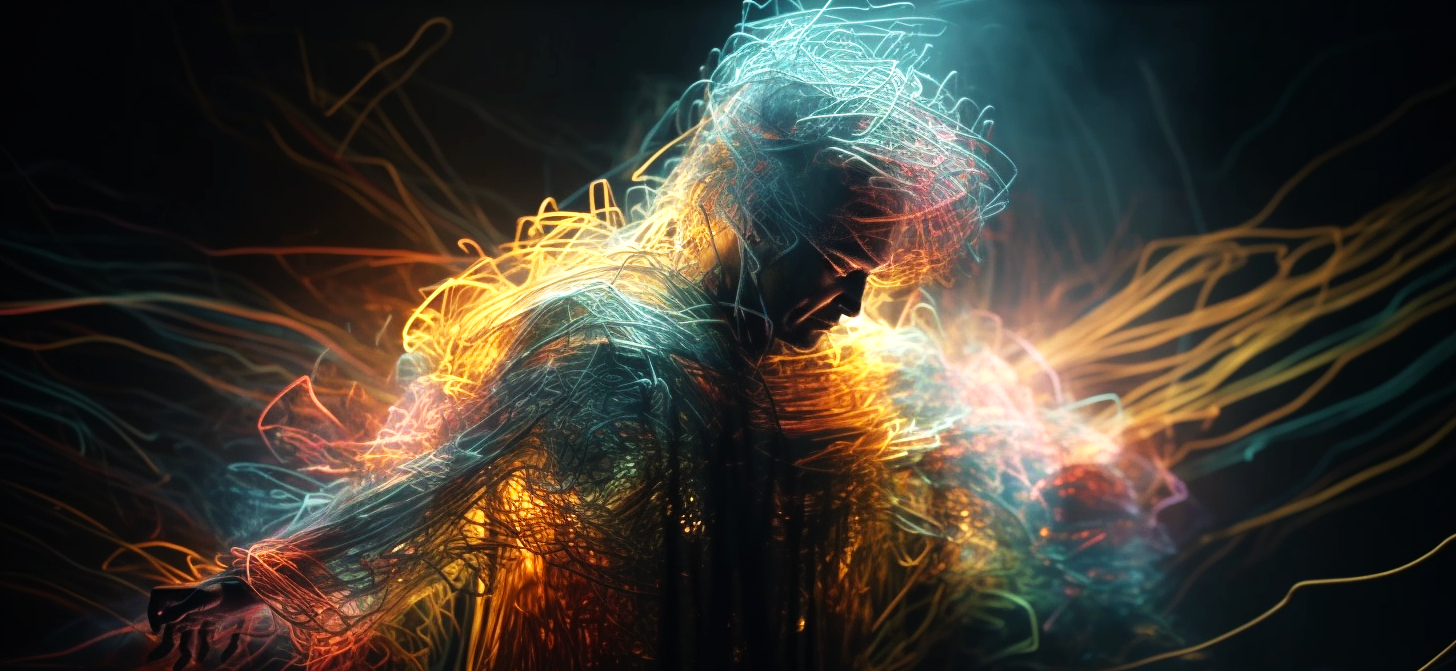
Recent advancements in AI, including upgrades in large language models and image generation, have showcased immense potential. However, odd behaviors in these systems, like Bing’s alter ego “Sydney” and eerie image generations in Stable Diffusion, raise concerns about machine super-intelligence. Nick Bostrom’s warnings about unregulated AI development emphasize the need for industry guardrails to ensure safe AI evolution and prevent uncontrollable advancements.